From Sparse Signal to Smooth Motion: Real-Time Motion Generation with Rolling Prediction Models
CVPR 2025
German Barquero1,2,3, Nadine Bertsch1, Manojkumar Marramreddy1, Carlos Chacón1, Filippo Arcadu1, Ferran Rigual1, Nicky Sijia He1, Cristina Palmero2,4, Sergio Escalera2,3, Yuting Ye1, and Robin Kips1
1Meta Reality Labs, 2Universitat de Barcelona, 3Computer Vision Center, 4King's College London
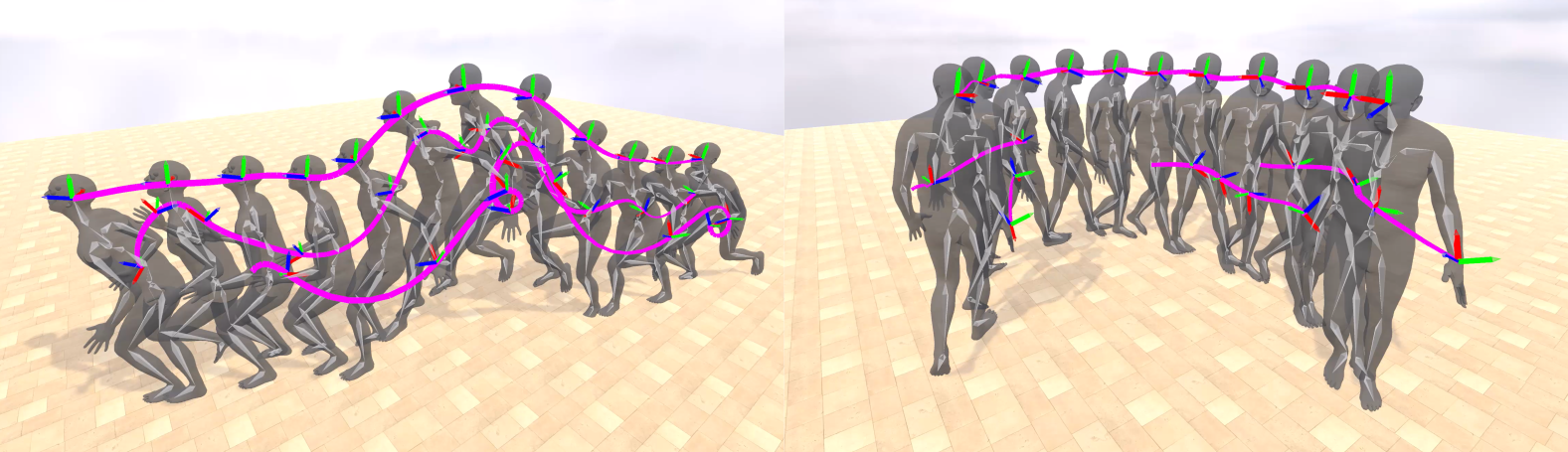
Abstract
In extended reality (XR), generating full-body motion of the users is important to understand their actions, drive their virtual avatars for social interaction, and convey a realistic sense of presence. While prior works focused on spatially sparse and always-on input signals from motion controllers, many XR applications opt for vision-based hand tracking for reduced user friction and better immersion. Compared to controllers, hand tracking signals are less accurate and can even be missing for an extended period of time. To handle such unreliable inputs, we present Rolling Prediction Model (RPM), an online and real-time approach that generates smooth full-body motion from temporally and spatially sparse input signals. Our model generates 1) accurate motion that matches the inputs (i.e., tracking mode) and 2) plausible motion when inputs are missing (i.e., synthesis mode). More importantly, RPM generates seamless transitions from tracking to synthesis, and vice versa. To demonstrate the practical importance of handling noisy and missing inputs, we present GORP, the first dataset of realistic sparse inputs from a commercial virtual reality (VR) headset with paired high quality body motion ground truth. GORP provides >14 hours of VR gameplay data from 28 people using motion controllers (spatially sparse) and hand tracking (spatially and temporally sparse). We benchmark RPM against the state of the art on both synthetic data and GORP to highlight how we can bridge the gap for real-world applications with a realistic dataset and by handling unreliable input signals. Our code, pretrained models, and GORP dataset are available in the project webpage.🌟 Key contributions 🌟
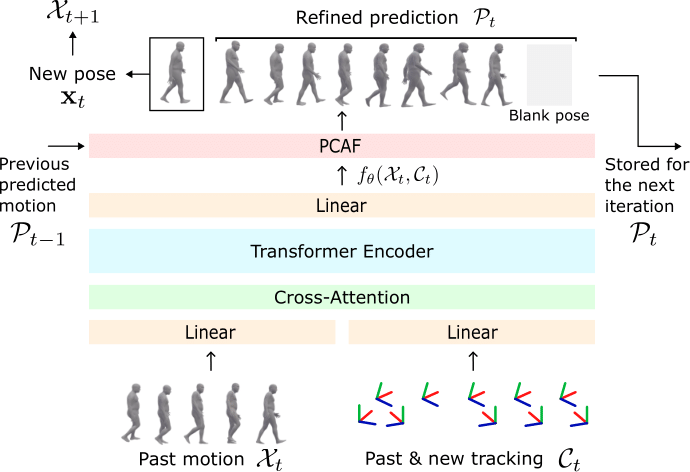
To evaluate our algorithm in realistic settings, we collected over 14 hours of VR gameplay data from Meta Quest 3, synchronized with high-precision ground truth body motion using an Optitrack system. Our proprietary synchronization methods achieve sub-millimeter accuracy. 28 participants played controller- and hand-tracking-based games designed to elicit diverse hand and arm movements, revealing tracking failures when hands or controllers leave the headset’s field of view. This is the first dataset combining real tracking signals with high-quality ground truth, highlighting practical challenges absent in synthetic data.
Our new GORP dataset exposes the field to difficult practical issues inexistent in synthetic data.
Our new GORP dataset exposes the field to difficult practical issues inexistent in synthetic data.
🎬 RPM vs. SOTA on the new GORP dataset
We observe how RPM synthesizes plausible motion during tracking signal losses (i.e., when the axis disappears). Once the tracking is recovered, RPM is the only method that generates a smooth transition towards matching it again. Other methods, instead, instantly snap the hands to the new tracking signal, breaking the continuity of the previous motion.
🔗 BibTeX
@inproceedings{barquero2025rolling,
title={From Sparse Signal to Smooth Motion: Real-Time Motion Generation with Rolling Prediction Models},
author={Barquero, German and Bertsch, Nadine and Marramreddy, Manojkumar and Chacón, Carlos and Arcadu, Filippo and Rigual, Ferran and He, Nicky and Palmero, Cristina and Escalera, Sergio and Ye, Yuting and Kips, Robin},
booktitle={Proceedings of the IEEE/CVF Conference on Computer Vision and Pattern Recognition},
year={2025},
}